13 Figure 3: Species composition
To illustrate how species composition changes with habitat types, we make a variety of plots:
- The top-5 taxonomic families per habitat type.
- The proportions of birds that belong to a selection of families that are representative for the entire Dutch landscape.
To quantify the necessary variables we rely mostly on the point-transect counts from Sovon as these cover all species (instead of the waterbird counts).
13.1 Processing environment
library(bioRad)
library(ggplot2)
library(dplyr)
library(tidyr)
library(stringr)
library(ggpointdensity)
library(patchwork)
library(GGally)
library(tibble)
library(ggpubr)
library(forcats)
We load the study PPI and proportions of taxonomic groups in the counts.
ppi <- readRDS("data/processed/composite-ppis/500m/201712312305.RDS")
wb_props <- readRDS("data/processed/sovon/wb_props.RDS")
ptt <- readRDS("data/processed/sovon/ptt.RDS")
ptt_props <- readRDS("data/processed/sovon/ptt_props.RDS")
ppi$data@data %>%
# left_join(wb_props, by = c("wb_area_nr" = "area_nr")) %>%
left_join(ptt_props, by = c("ptt_route" = "route")) %>%
filter(coverage > 0,
class != 1,
total_biomass > 0,
dist_radar < 66000,
urban < 0.1) %>%
identity() -> data
We select only those PPI pixels (and their corresponding counts) which are covered for >99% by a single habitat type.
landuse_limit <- 0.99
13.2 Characteristic families
Geese, ducks, pigeons, thrushes, tits, waders, crows and finches represent a substantial part of the Dutch avifauna across a large range of body sizes. We select these to illustrate how the taxonomic proportions change with habitats.
selected_families <- c("Geese", "Ducks", "Pigeons", "Thrushes", "Tits", "Waders", "Crows", "Finches")
data %>%
dplyr::select(all_of(c(colnames(ptt_props)[3:45], "semiopen", "forests", "wetlands", "waterbodies", "agricultural"))) %>%
pivot_longer(cols = all_of(colnames(ptt_props)[3:45]), names_to = "family", values_to = "family_prop") %>%
pivot_longer(cols = all_of(c("agricultural", "semiopen", "forests", "wetlands", "waterbodies")), names_to = "landuse", values_to = "landuse_prop") %>%
drop_na() %>%
filter(family %in% selected_families,
landuse_prop >= landuse_limit) %>%
group_by(family, landuse) %>%
summarise(mean_family_prop = mean(family_prop), .groups = "drop_last") %>%
pivot_wider(names_from = landuse, values_from = mean_family_prop) %>%
mutate(family_sc = case_when(family == "Geese" ~ "Anatidae",
family == "Ducks" ~ "Anatinae",
family == "Pigeons" ~ "Columbidae",
family == "Thrushes" ~ "Turdidae",
family == "Tits" ~ "Paridae",
family == "Waders" ~ "Charadrii",
family == "Crows" ~ "Corvidae",
family == "Finches" ~ "Fringillidae")) %>%
identity() -> characteristic_families
characteristic_families
family | agricultural | forests | semiopen | waterbodies | wetlands | family_sc |
---|---|---|---|---|---|---|
Crows | 0.0619286 | 0.1373295 | 0.0740222 | 0.0182881 | 0.0407936 | Corvidae |
Ducks | 0.1508770 | 0.0233594 | 0.0726795 | 0.3419478 | 0.2416624 | Anatinae |
Finches | 0.0152677 | 0.1314283 | 0.0628657 | 0.0062778 | 0.0102175 | Fringillidae |
Geese | 0.2946680 | 0.0281144 | 0.0384954 | 0.3065711 | 0.0666727 | Anatidae |
Pigeons | 0.0508753 | 0.0549949 | 0.0921633 | 0.0077864 | 0.0028696 | Columbidae |
Thrushes | 0.0228345 | 0.0957379 | 0.0658859 | 0.0068034 | 0.0121501 | Turdidae |
Tits | 0.0084950 | 0.2470226 | 0.0778224 | 0.0051277 | 0.1186220 | Paridae |
Waders | 0.0953540 | 0.0006251 | 0.0310129 | 0.0873656 | 0.2058158 | Charadrii |
13.3 Mean habitat biomass and weight
We calculate the mean biomass for all areas covered for >99% by a single habitat type and calculate mean bird weight for these areas from the point-transect counts.
data %>%
dplyr::select(all_of(c("total_biomass", "ptt_weighted_mean_weight", "semiopen", "forests", "wetlands", "waterbodies", "agricultural"))) %>%
pivot_longer(cols = all_of(c("semiopen", "forests", "wetlands", "waterbodies", "agricultural")), names_to = "landuse", values_to = "proportion") %>%
filter(proportion >= landuse_limit) %>%
mutate(total_biomass = total_biomass / 1000) %>%
group_by(landuse) %>%
summarise(mean_biomass = mean(total_biomass),
mean_weight = mean(ptt_weighted_mean_weight, na.rm = TRUE),
.groups = "drop_last") %>%
arrange(desc(mean_weight)) %>%
identity() -> habitat_mass
habitat_mass
landuse | mean_biomass | mean_weight |
---|---|---|
waterbodies | 100.098362 | 1367.6172 |
agricultural | 133.003369 | 1256.0901 |
wetlands | 213.892151 | 866.0075 |
semiopen | 31.895918 | 742.2743 |
forests | 4.002946 | 246.8706 |
13.4 Sorting of families by weight
Now we sort families according to weight
ptt %>%
distinct(species, .keep_all = TRUE) %>%
group_by(familyvernacular) %>%
summarise(mean_weight = mean(mean_weight), .groups = "drop_last") %>%
filter(familyvernacular %in% selected_families) %>%
arrange(desc(mean_weight)) %>%
rowid_to_column()-> family_weights
characteristic_families %>%
left_join(family_weights, by = c("family" = "familyvernacular")) %>%
arrange(desc(mean_weight)) -> characteristic_families
characteristic_families$rank <- factor(characteristic_families$mean_weight, ordered = TRUE, levels = characteristic_families$mean_weight)
13.5 Prepare plot
And plot a simple barplot figure.
characteristic_families %>%
pivot_longer(cols = !c("family", "rowid", "mean_weight", "rank", "family_sc"), names_to = "landuse", values_to = "family_prop") -> cf_long
colors <- c("urban" = "#94346E", "agricultural" = "#73AF48", "semiopen" = "#EDAD08", "forests" = "#0F8554", "wetlands" = "#38A6A5", "waterbodies" = "#1D6996",
"dist_urban" = "#94346E", "total_biomass" = "#CC503E", "dist_radar" = "#666666")
landuse_names <- c("agricultural" = "Agricultural", "semiopen" = "Semi-open", "forests" = "Forests", "wetlands" = "Wetlands", "waterbodies" = "Water bodies")
plot_family_proportions <- function(props, which) {
landuse_labeller <- function(variable, value) {
label <- paste0(landuse_names[value], " ", round(habitat_mass[habitat_mass$landuse == which, ]$mean_weight, digits = 0), "g")
return(label)
}
proportion_labeller <- function(value) paste0(value * 100, "%")
props %>%
filter(landuse == which) %>%
ggplot() +
geom_col(aes(x = family_prop, y = reorder(family, mean_weight), alpha = family_prop), fill = colors[which]) +
labs(x = "Proportion of birds", y = "Taxonomic group") +
coord_cartesian(expand = FALSE, xlim = c(0, ceiling(max(cf_long$family_prop) * 100) / 100)) +
scale_x_continuous(breaks = c(0, 0.1, 0.2, 0.3), labels = proportion_labeller) +
scale_alpha_continuous(range = c(0.4, 1)) +
facet_wrap(vars(landuse), strip.position = "top") +
theme_classic(base_size = 12) +
theme(legend.position = "none",
axis.title.y = element_blank(),
strip.background = element_blank(),
strip.text.x = element_blank())
}
landuses <- c("agricultural", "semiopen", "forests", "wetlands", "waterbodies") # Same order as model outputs
plots <- mapply(function(landuses) { plot_family_proportions(cf_long, landuses) }, landuses = landuses,
SIMPLIFY = FALSE)
# characteristic_families$title <- "Mean weight (g)"
# ggplot(characteristic_families) +
# geom_col(aes(x = mean_weight, y = reorder(family, mean_weight))) +
# labs(x = "Mean weight (g)", y = "Taxonomic group") +
# coord_cartesian(expand = FALSE) +
# scale_x_continuous(breaks = c(0, 1000, 2000, 3000)) +
# facet_wrap(vars(title), strip.position = "top") +
# theme_classic(base_size = 12) +
# theme(legend.position = "none",
# axis.title.y = element_blank(),
# strip.background = element_blank(),
# strip.text.x = element_text(hjust = 0, size = 12, family = "Helvetica", face = "bold")) -> plots[[6]]
p <- wrap_plots(plots, ncol = 1)
p
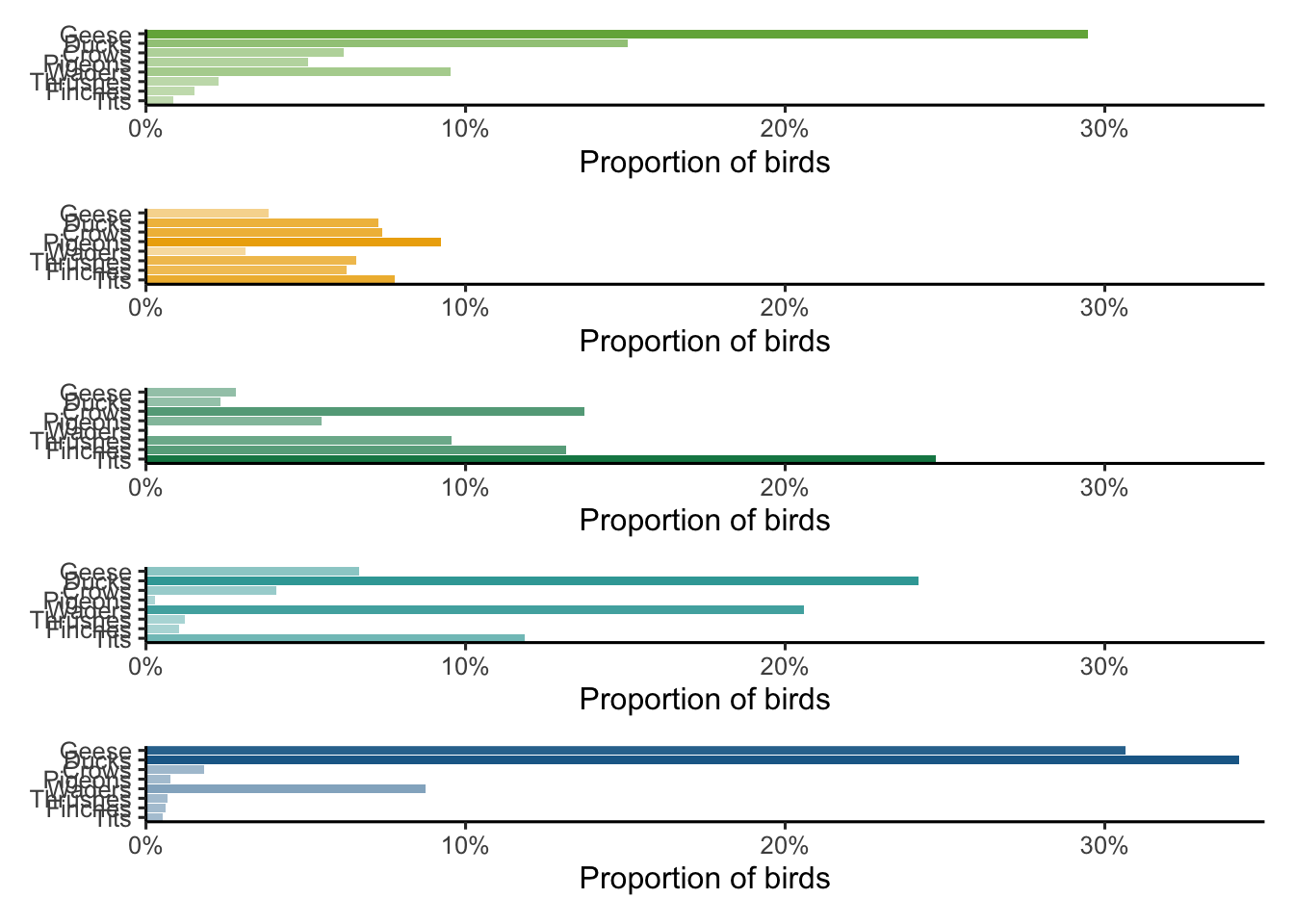
p_models <- readRDS("data/plots/paper/fig_landscapes.RDS")
p_models[[1]] + p_models[[2]] + p_models[[3]] + p_models[[4]] + p_models[[5]] +
plots[[1]] + plots[[2]] + plots[[3]] + plots[[4]] + plots[[5]] +
plot_layout(widths = c(1, 1), nrow = 5, byrow = FALSE) &
theme(axis.text.x = element_text(size = 10),
axis.title.x = element_text(size = 12),
axis.text.y = element_text(size = 10)) -> p_landscape_characterisation
ggsave(filename = "data/plots/paper/fig2_landscape.pdf", plot = p_landscape_characterisation, width = 14, height = 25, dpi = 300, units = "cm")
p_landscape_characterisation
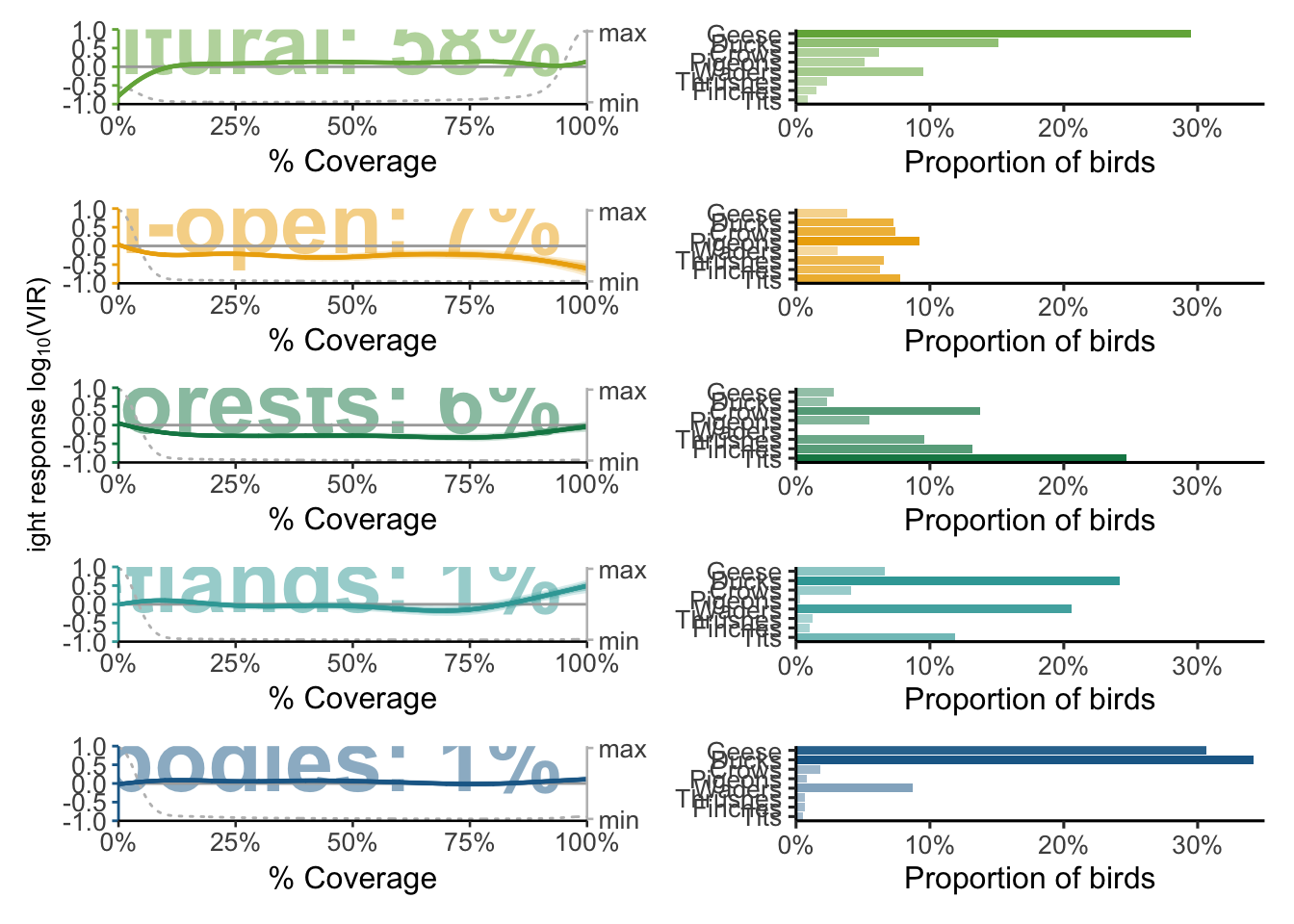
widths <- c(1, 1)
(p_models[[1]] / plots[[1]] * theme(axis.text.x = element_blank(), axis.title.x = element_blank(), axis.text.y = element_text(size = 10)) +
plot_layout(widths = widths, ncol = 2)) /
(p_models[[2]] / plots[[2]] * theme(axis.text.x = element_blank(), axis.title.x = element_blank(), axis.text.y = element_text(size = 10)) +
plot_layout(widths = widths, ncol = 2)) /
(p_models[[3]] / plots[[3]] * theme(axis.text.x = element_blank(), axis.title.x = element_blank(), axis.text.y = element_text(size = 10)) +
plot_layout(widths = widths, ncol = 2)) /
(p_models[[4]] / plots[[4]] * theme(axis.text.x = element_blank(), axis.title.x = element_blank(), axis.text.y = element_text(size = 10)) +
plot_layout(widths = widths, ncol = 2)) /
(p_models[[5]] / plots[[5]] * theme(axis.text.x = element_text(size = 10), axis.title.x = element_text(size = 12), axis.text.y = element_text(size = 10)) +
plot_layout(widths = widths, ncol = 2)) -> p_landscape_characterisation
p_landscape_characterisation
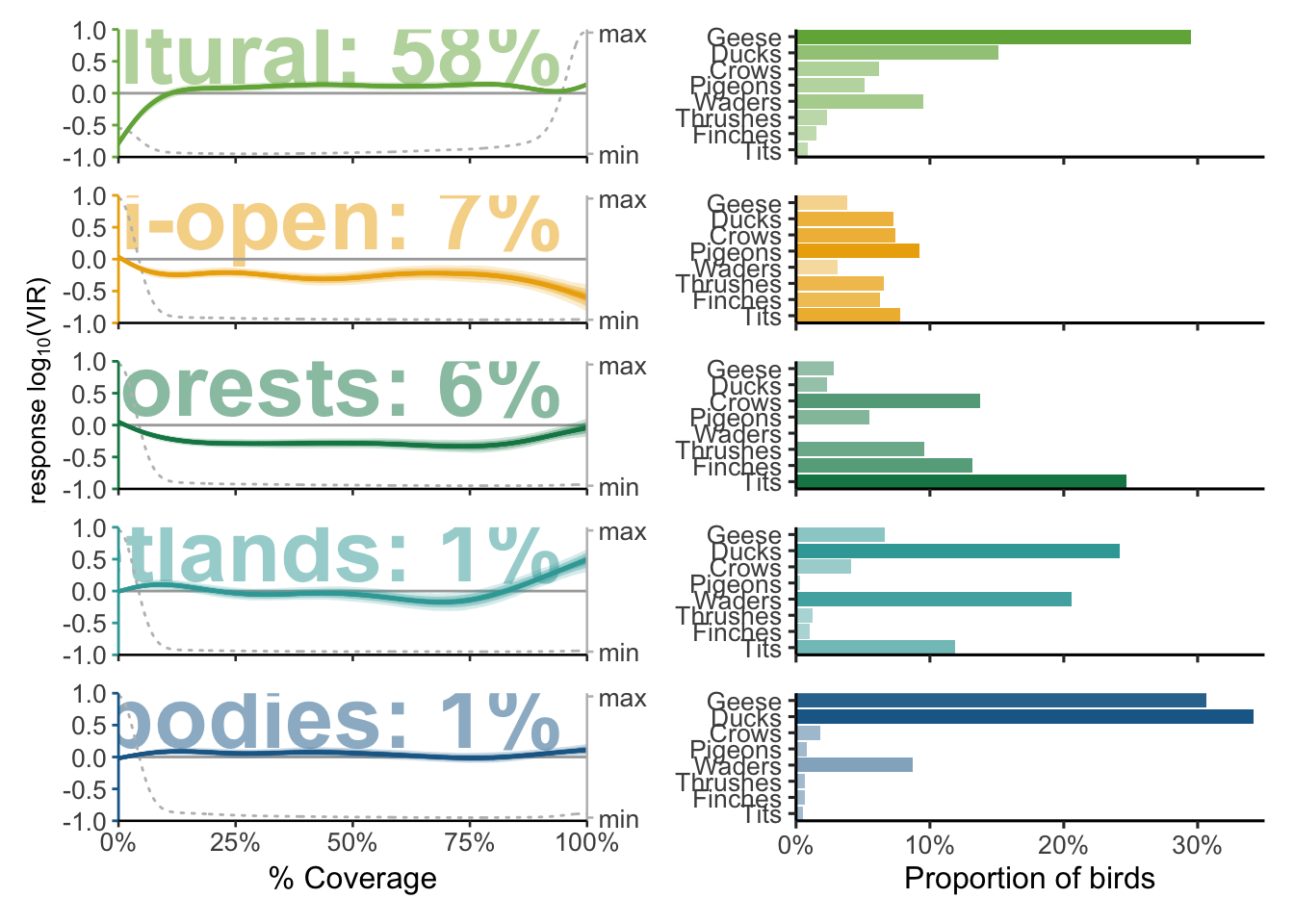
And we save it as .pdf for editing in Illustrator.
ggsave(filename = "data/plots/paper/fig2_landscape.pdf", plot = p_landscape_characterisation, width = 12, height = 18, dpi = 300, units = "cm")